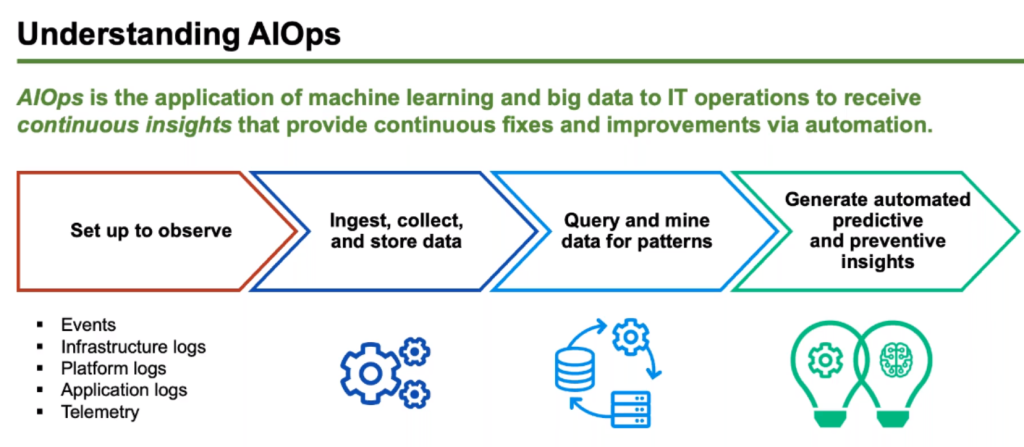
Here are some of the best practices for AIOps:
- Start with a clear understanding of your goals and objectives. What do you want to achieve with AIOps? Do you want to improve efficiency, reduce costs, or improve performance? Once you know your goals, you can start to develop a plan to achieve them.
- Assess your current IT environment. What data sources do you have available? What tools are you using? What skills do your team have? This will help you to determine what AIOps solution is right for you.
- Choose the right AIOps solution. There are a number of different AIOps solutions available. The best solution for you will depend on your specific needs and requirements. When selecting an AIOps solution, consider the following factors:
- The size and complexity of your IT environment
- The specific problems that you want to solve
- Your budget
- The level of support that you require
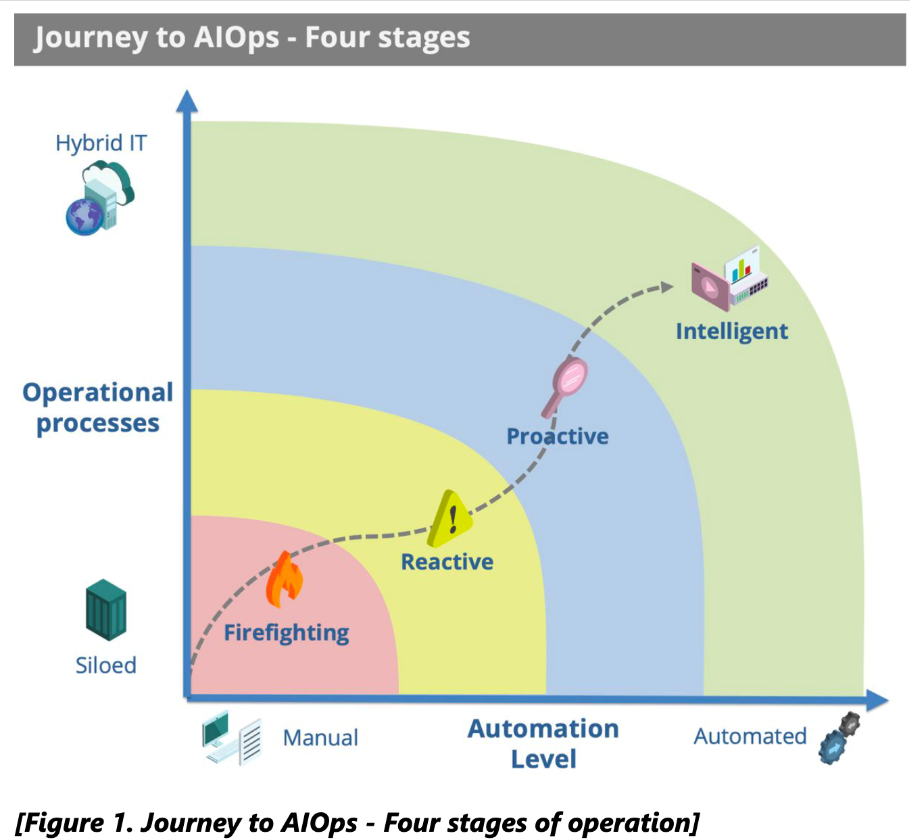
- Gather the right data. The data that you gather will be used to train the AI models. So it is important to gather the right data. This data can come from a variety of sources, such as monitoring tools, ticketing systems, and event logs.
- Train the models correctly. The models need to be trained correctly in order to be effective. This involves using the right data, the right algorithms, and the right training parameters.
- Deploy the models in production. Once the models are trained, they need to be deployed in production. This involves integrating the models with your IT operations tools and processes.
- Monitor the results. Once the models are deployed, you need to monitor the results to ensure that they are working as expected. This includes monitoring the accuracy of the models, the performance of the algorithms, and the impact of AIOps on IT operations.
- Iteratively improve the models. AIOps is a continuously evolving field. As you gain more experience with AIOps, you will need to iteratively improve the models. This may involve retraining the models, adjusting the algorithms, or adding new features.
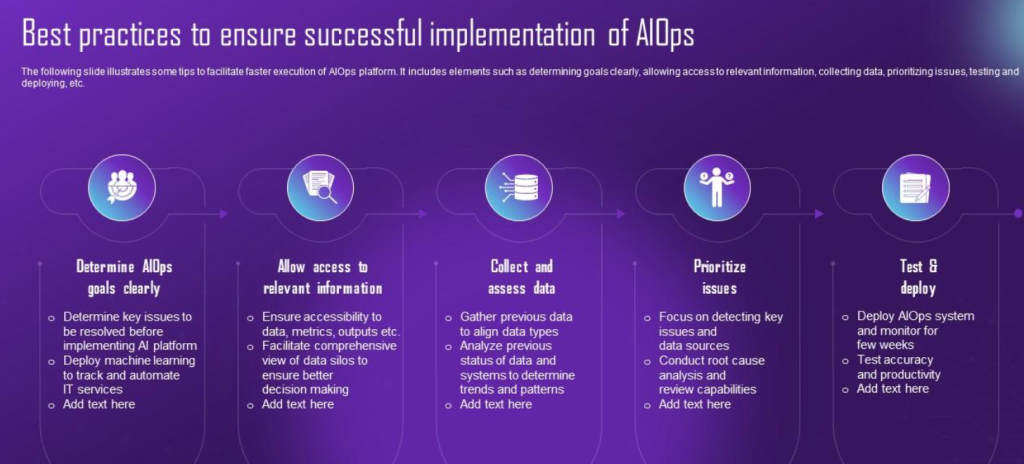
By following these best practices, you can implement AIOps in your organization and start to reap the benefits.Here are some additional tips for implementing AIOps:
- Start small and scale up as needed. Don’t try to implement AIOps all at once. Start with a small pilot project and then scale up as you gain more experience.
- Get buy-in from stakeholders. AIOps is a complex undertaking, so it’s important to get buy-in from stakeholders from the beginning. This will help to ensure that the project is successful.
- Communicate with your team. As you implement AIOps, it’s important to communicate with your team about what you’re doing and why. This will help to ensure that everyone is on the same page and that there are no surprises.
- Be patient. AIOps is a journey, not a destination. It takes time to gather data, train models, and deploy solutions. Be patient and persistent, and you will eventually see the benefits of AIOps.
Best Practices in Implementing AIOps
Getting the most out AIOps requires that DevOps engineers implement the following best practices.
Proper Data Management
DevOps engineers must be aware of the fact that ill-managed data always gives rise to undesired output and affects the decisions made when using the output. For top-notch outcomes, DevOps engineers should ensure the gathered data is properly cleaned, sorted, and classified. Performing these actions makes the process of browsing through large datasets easier than you might expect.
Proper Data Security
The security of user data is something firms can’t play with, as data protection regulation agencies can impose fines. Thus, the DevOps team must ensure they properly safeguard data within its control to avoid data breaches. This is especially important during the process of transmitting data into AI-enabled analysis platforms.
Proper Task Hierarchy Assignment
This is another best practice engineers should observe when implementing AIOps. It gives team members the ability to easily have an overview of all tasks and decide which they should attend to first.Bearing this in mind, DevOps engineers should break down large tasks into smaller tasks, and develop a kind of hierarchy of priority for these tasks into the ML model. ML then takes it from there and does the rest. For instance, ML notify developers when tasks are due, who should handling a task, and so on.
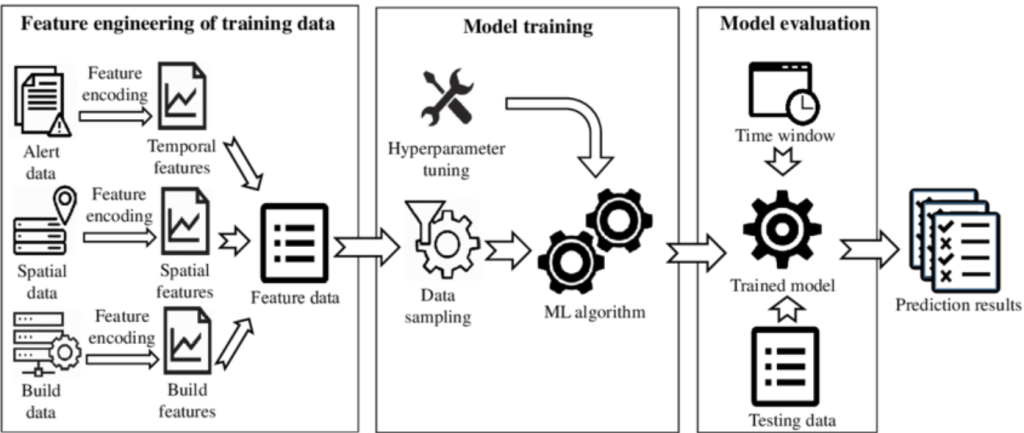
Proper Use of Available AI APIs
AIOps is an amalgam of whatever artificial intelligence can offer to improve the productivity of IT operations. Therefore, the DevOps team should be on the lookout for great AI-enabled APIs capable of improving whatever task they have to accomplish.