Upgrade & Secure Your Future with DevOps, SRE, DevSecOps, MLOps!
We spend hours scrolling social media and waste money on things we forget, but wonโt spend 30 minutes a day earning certifications that can change our lives.
Master in DevOps, SRE, DevSecOps & MLOps by DevOps School!
Learn from Guru Rajesh Kumar and double your salary in just one year.
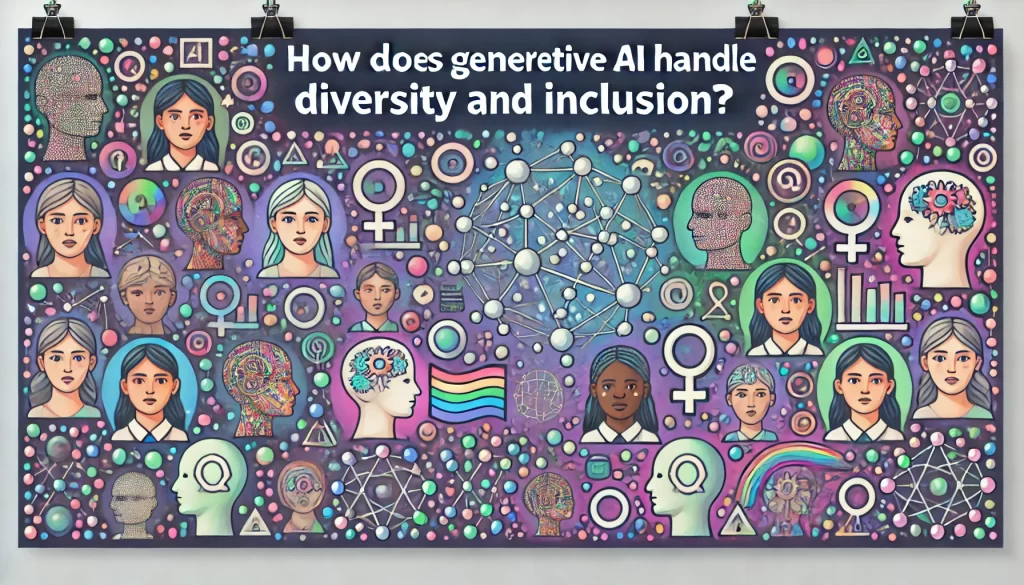
Generative AI has transformed how we create content, offering the power to generate text, images, videos, and more. However, as with any technology, generative AI needs to promote diversity and inclusion, ensuring that the content it produces represents and respects various cultures, identities, and perspectives. Failing to address these factors can result in biased or harmful outputs that reinforce stereotypes or exclude underrepresented groups. Hereโs a look at how generative AI handles diversity and inclusion, the challenges it faces, and steps being taken to make AI-generated content fairer and more inclusive.
Understanding Diversity and Inclusion in AI
Diversity in AI refers to the modelโs ability to represent a wide range of identities, perspectives, and backgrounds accurately. Inclusion ensures that AI systems do not marginalize any group and instead celebrate diversity by producing content that resonates with all users. For generative AI, this involves avoiding stereotypes, reflecting different cultural contexts, and generating outputs that are respectful and representative of all people.
Example: In image generation, diversity and inclusion mean producing images that represent various ethnicities, ages, body types, genders, and lifestyles. In text generation, it involves generating inclusive language and avoiding harmful stereotypes.
Challenges of Promoting Diversity and Inclusion in Generative AI
Despite advancements, promoting diversity and inclusion in generative AI is challenging due to several factors:
- Bias in Training Data: Generative AI models are often trained on large datasets collected from the internet, where biases may exist in language, imagery, and representation. For instance, certain demographic groups may be underrepresented, leading models to generate outputs that reflect limited perspectives.
- Reinforcement of Stereotypes: If the data reflects societal biases or stereotypes, the model may unintentionally produce biased outputs, reinforcing these patterns. For example, a model trained on certain types of images might consistently associate specific professions or roles with certain genders or ethnicities.
- Technical Complexity: Ensuring fair representation in generative AI is technically complex, as models need to recognize and accurately represent diversity across multiple attributes (e.g., gender, culture, age) without reducing these to stereotypes or clichรฉs.
- Subjective Nature of Inclusivity: What constitutes โinclusiveโ or โrespectfulโ content may vary across cultures and individuals, making it challenging to ensure that AI outputs meet universal standards.
Strategies for Enhancing Diversity and Inclusion in Generative AI
To address these challenges, researchers and developers are implementing various strategies to make generative AI outputs more inclusive:
a. Diverse and Balanced Training Data
- Goal: Use datasets that represent a wide range of ethnicities, genders, cultures, and other aspects of human diversity.
- Implementation: By curating datasets to include a balanced representation of different groups, developers can ensure that models learn to generate content that reflects global diversity.
- Example: Including images and language from different cultural contexts to help AI systems recognize and respect regional diversity.
b. Bias Detection and Mitigation
- Goal: Identify and reduce biases in model outputs, ensuring fairness and inclusivity.
- Implementation: Researchers use techniques like adversarial testing and bias detection algorithms to evaluate model outputs, identifying patterns that reflect biases and addressing them.
- Example: Running test cases to ensure that text models donโt associate certain professions exclusively with one gender or that image models represent diverse body types in fitness content.
c. Regular Audits and Human Oversight
- Goal: Conduct regular checks on model outputs to identify any potential biases and make adjustments accordingly.
- Implementation: By involving diverse teams in auditing model outputs, companies can gather feedback on the inclusivity and cultural sensitivity of generated content.
- Example: A diverse panel of reviewers evaluates AI-generated content to ensure it meets inclusivity standards, identifying areas where adjustments may be needed.
d. Incorporating Inclusive Language and Imagery Guidelines
- Goal: Set clear guidelines that emphasize inclusivity and prevent the reinforcement of stereotypes.
- Implementation: Developers incorporate specific constraints into the modelโs training process or use post-processing rules to avoid language or imagery that might exclude or misrepresent certain groups.
- Example: Text models can be guided to use gender-neutral language or avoid terms that might perpetuate stereotypes, ensuring a respectful tone in generated content.
e. User Control and Customization
- Goal: Allow users to customize outputs to match their cultural context or preferences, making generative AI more adaptable to individual needs.
- Implementation: Providing customization options where users can specify characteristics such as cultural tone, language style, or demographic representation.
- Example: An AI image generator might let users specify attributes like age range, clothing style, or skin tone to ensure outputs align with the userโs intent.
Examples of Diversity and Inclusion in Generative AI Applications
Several applications of generative AI illustrate how diversity and inclusion are integrated:
- Image Generation: Tools like DALL-E and Midjourney are evolving to generate images that represent diverse demographics. Developers are continually refining these models to produce visuals that showcase people of different ethnicities, body types, and ages.
- Language Models: Language models like ChatGPT and Jasper are regularly updated to avoid generating biased or exclusionary language. By incorporating diverse text sources and setting guidelines for inclusive language, these models are better at generating content that resonates across cultures.
- Virtual Avatars and Video Generation: Platforms like Synthesia allow users to select avatars that represent different ethnicities, genders, and ages, ensuring that businesses can create inclusive, relatable content for their audiences.
Future Directions for Diversity and Inclusion in Generative AI
As generative AI technology advances, developers and researchers are pursuing ways to strengthen its diversity and inclusivity:
- Dynamic Bias Correction: Developing models that can adapt based on feedback, identifying and mitigating biases as they arise. This would allow generative AI to correct itself and become more inclusive over time.
- Community-Driven Datasets: Involving communities in curating training data to ensure representation of diverse cultural and regional contexts, reducing reliance on limited or biased data sources.
- Inclusive AI Standards: Creating industry-wide standards and guidelines for inclusivity in generative AI, helping developers prioritize diversity and inclusion in all stages of model development.
- Transparent AI: Making AI systems more transparent by providing users with information on how models were trained and how they address diversity and inclusion. This can help build trust and accountability in AI applications.
Leave a Reply