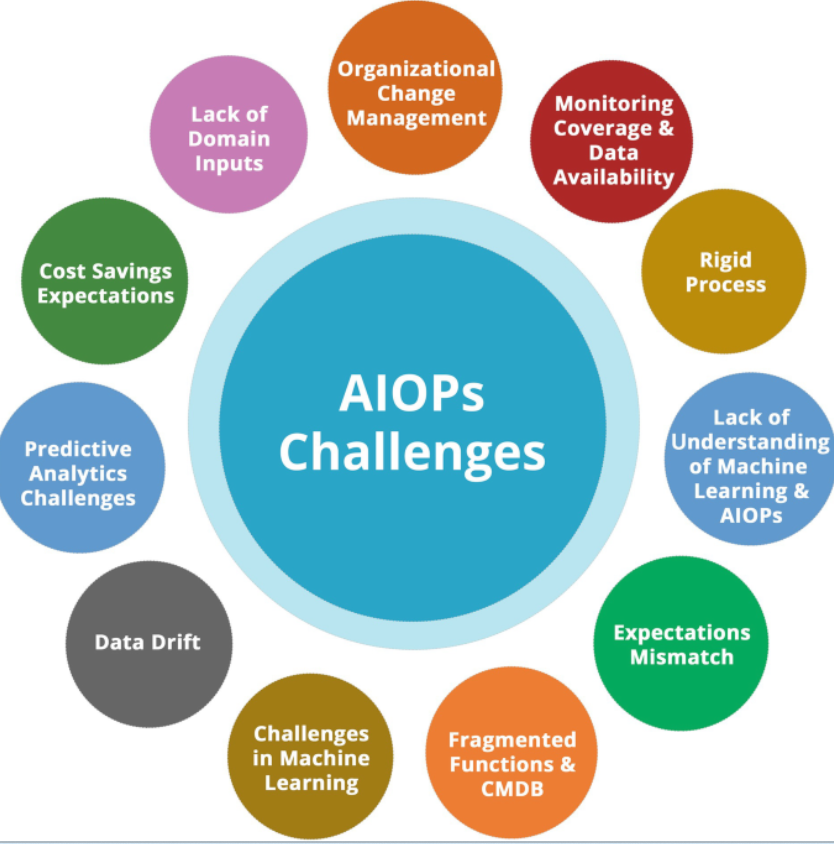
While AIOps (Artificial Intelligence for IT Operations) offers numerous benefits, it also comes with its own set of challenges and considerations. Here are some of the challenges associated with implementing AIOps:
- Data Quality and Complexity:
- AIOps relies heavily on accurate and high-quality data for effective analysis and decision-making. Poor data quality, incomplete data, or data from disparate sources can lead to inaccurate insights and recommendations.
- Managing the complexity of data from various sources, formats, and sources can be challenging.
- Data Volume and Scalability:
- In large and dynamic IT environments, the sheer volume of data generated can overwhelm AIOps systems. Processing and analyzing massive amounts of data in real-time requires significant computational resources.
- Algorithm Selection and Tuning:
- Choosing the right machine learning algorithms and models for specific use cases can be complex. Proper tuning and optimization of algorithms are essential for accurate predictions and analysis.
- Model Bias and Accuracy:
- Machine learning models used in AIOps can exhibit bias or make inaccurate predictions if not trained properly or if the training data is biased. Ensuring fairness and accuracy is crucial to reliable insights.
- Data Privacy and Security:
- Handling sensitive data within AIOps systems raises concerns about data privacy and security. Organizations need to implement robust security measures to protect sensitive information.
- Integration and Adoption:
- Integrating AIOps into existing IT infrastructure and workflows can be challenging. Resistance to change and lack of understanding among IT teams can hinder successful adoption.
- Skill Gap:
- Implementing AIOps requires specialized skills in data science, machine learning, and AI. Organizations may need to invest in training or hire experts to effectively use AIOps technologies.
- False Positives and Negatives:
- AIOps systems may generate false alerts or overlook actual issues, leading to wasted resources or missed opportunities to address real problems.
- Change Management:
- Transitioning from traditional IT operations to AIOps may require changes in processes, roles, and responsibilities. Managing this transition effectively can be challenging.
- Continuous Learning and Adaptation:
- AIOps systems need ongoing training and updates to adapt to changes in the IT environment. Without continuous learning, the accuracy of predictions can decline over time.
- Vendor Landscape and Tool Selection:
- Choosing the right AIOps tools and vendors can be challenging due to the evolving nature of the technology and the multitude of options available.
- Cost and Return on Investment (ROI):
- Implementing AIOps involves costs for infrastructure, software, training, and personnel. Organizations need to assess the potential ROI and long-term benefits.
Despite these challenges, many organizations are finding ways to overcome them and leverage AIOps to streamline IT operations, improve efficiency, and enhance overall IT service quality. Careful planning, proper data management, and a clear understanding of organizational needs are key to successful AIOps implementation.
Here are some of the challenges of AIOps:
- Data quality: The quality of the data used by AIOps solutions is critical to their success. If the data is not accurate or complete, the models built by AIOps solutions will be inaccurate. This can lead to false positives and negatives, which can waste time and resources.
- Complexity: AIOps solutions can be complex to implement and manage. This is because they typically involve a lot of different data sources, algorithms, and models. This can make it difficult to understand how the solutions work and to troubleshoot problems.
- Security and privacy: AIOps solutions can collect a lot of sensitive data about IT systems and operations. This data needs to be protected from unauthorized access and disclosure. This can be a challenge, as AIOps solutions often collect data from a variety of different sources, including cloud-based and on-premises systems.
- Bias: AIOps solutions can be biased, which can lead to unfair or inaccurate results. This can happen if the data used to train the models is not representative of the real world.
- Interpretability: It can be difficult to understand how AIOps solutions make decisions. This can make it difficult to trust the solutions and to use them effectively.
- Vendor lock-in: AIOps solutions are often proprietary, which can make it difficult to switch vendors if needed. This can be a problem if the vendor goes out of business or if the solution does not meet the organization’s needs.
Despite these challenges, AIOps is a promising technology that has the potential to revolutionize IT operations. As the technology matures and the challenges are addressed, AIOps is likely to become an essential tool for organizations of all sizes.Here are some of the things that organizations can do to address the challenges of AIOps:
- Invest in data quality: Organizations should invest in data quality initiatives to ensure that the data used by AIOps solutions is accurate and complete. This can involve cleaning the data, removing outliers, and normalizing the data.
- Simplify the solution: Organizations should choose AIOps solutions that are easy to implement and manage. This can involve choosing solutions that are integrated with existing IT systems and that use simple, easy-to-understand algorithms.
- Protect data: Organizations should take steps to protect the data collected by AIOps solutions. This can involve using encryption, access controls, and auditing.
- Address bias: Organizations should address bias in AIOps solutions by using techniques such as data augmentation and algorithm selection.
- Make the solution interpretable: Organizations should make AIOps solutions interpretable by using techniques such as explainability AI and machine learning interpretability.
- Avoid vendor lock-in: Organizations should choose AIOps solutions that are open source or that allow for easy integration with other solutions.
By addressing these challenges, organizations can make AIOps a valuable tool for improving IT operations.