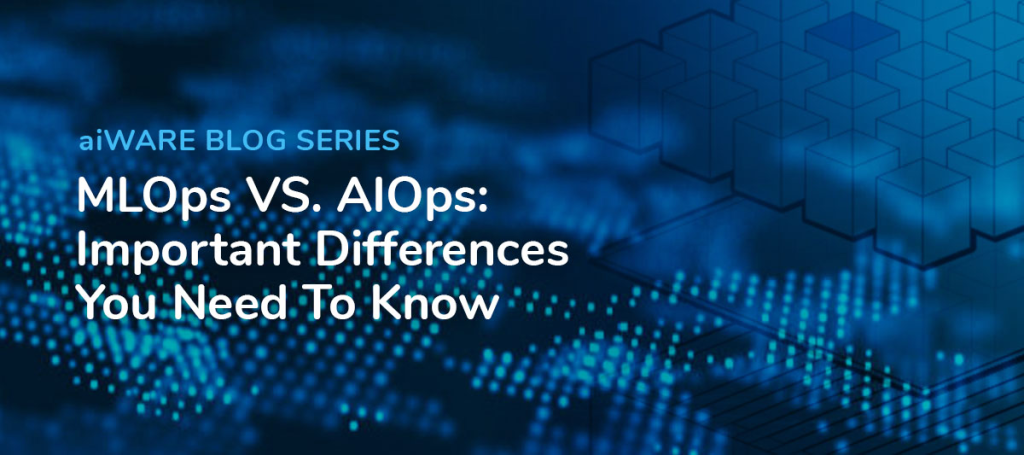
When it comes to managing and scaling machine learning (ML) and artificial intelligence (AI) applications, two terms that often come up are AIOps and MLOps. While these two terms may sound similar, they actually refer to very different things. In this blog post, we’ll explore the major differences between AIOps vs MLOps.
AIOps: The Basics
AIOps stands for Artificial Intelligence for IT Operations. As the name suggests, AIOps is all about using AI and ML to optimize IT operations. This includes tasks like:
- Monitoring system performance
- Predicting and preventing outages
- Automating routine tasks
- Analyzing log data
AIOps is all about using AI and ML to help IT teams work smarter, not harder. By automating routine tasks and streamlining workflows, AIOps can help organizations save time and reduce the risk of human error.
MLOps: The Basics
MLOps stands for Machine Learning Operations. MLOps is all about managing and scaling ML applications. This includes tasks like:
- Building and training ML models
- Deploying ML models to production
- Monitoring and optimizing ML models
- Managing data pipelines
MLOps is all about ensuring that ML applications are reliable, scalable, and efficient. By managing data pipelines and automating tasks like model deployment and monitoring, MLOps can help organizations get the most out of their ML investments.
The Major Differences between AIOps vs MLOps
While AIOps and MLOps share some similarities, there are several key differences between the two.
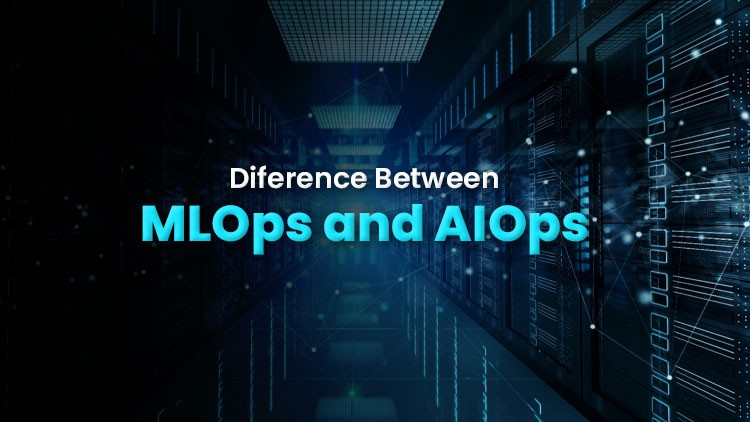
Focus
The biggest difference between AIOps and MLOps is their focus. AIOps is all about optimizing IT operations, while MLOps is all about managing and scaling ML applications.
Tools
Another major difference between AIOps and MLOps is the tools they use. AIOps relies heavily on log analysis tools, while MLOps relies on tools like Jupyter Notebooks, TensorFlow, and PyTorch.
Data
AIOps and MLOps also differ in the types of data they work with. AIOps typically works with log data, while MLOps works with larger datasets of structured and unstructured data.
Team
Finally, AIOps and MLOps require different skill sets. AIOps requires expertise in IT infrastructure and operations, while MLOps requires expertise in data science and ML.
Conclusion
In conclusion, while AIOps and MLOps may sound similar, they are actually quite different. AIOps focuses on optimizing IT operations using AI and ML, while MLOps focuses on managing and scaling ML applications. By understanding the differences between these two terms, organizations can make informed decisions about which approach is best for their needs.